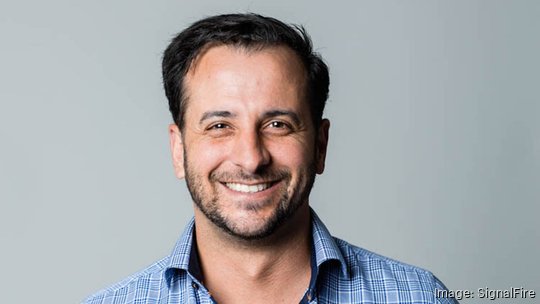
While artificial intelligence has exploded into the zeitgeist over the past several months, a San Francisco venture capital firm has been building an AI-powered database for a decade and uses it to inform its investing strategy.
SignalFire was co-founded in 2013 by partners Chris Farmer and Ilya Kirnos, who had previously worked at General Catalyst and Bessemer Venture Partners, and Google, respectively.
Aiming to harness the power of AI for making investment decisions as venture capitalists, they created a proprietary database called Beacon. Over the years, they also added capabilities to help their portfolio companies find and recruit talent.
It costs at least $10 million annually to maintain the database, they said. In the firm's early days, they licensed access to third parties to recoup some of the costs of building and maintaining the database, but they no longer do so.
Beacon now tracks 495 million employees and 80 million companies, mostly across the U.S. In February, they announced closing on $900 million in new capital commitments to invest across various funds, with an eye towards seeking out AI-forward companies in a variety of industries including healthcare, cybersecurity and developer tools.
I recently spoke with Farmer and Kirnos, in separate interviews, about why they created Beacon and how it works. The following transcript has been edited and condensed for clarity.
What is Beacon and why did you create it?
Farmer: The genesis of Beacon goes back to something like 2007. When I was at Bessemer Venture Partners, I was covering mobile from wireless for them when the App Store came out. And I'm like, it's a little hard to eyeball the App Store, there's a lot going on there. There's this thing called an algorithm. A bunch of years later, I started tweaking and evolving these things. And as a VC, there's always paranoia that you're missing out on the right party, where the next Mark Zuckerberg may be, or Larry Page, that you're supposed to meet at a demo day. I wanted to build a platform that was less about serendipity.
It's almost like creating a credit report for someone. We create a mosaic around what is something like 80 million entities, some subset of which become companies. It may start as an open-source project or a Web3 project or a founder — they might never start a company or they may never even incorporate but they may put founder somewhere on the internet about themselves. We create records of all of these types of things around the world.
Kirnos: The irony of our industry is, venture capitalists invest in these cutting-edge technology companies, right? We're always looking for technology driven disruption. And yet, when you look at venture capital itself as an industry, it's quite tech deficient. VCs love to talk about applying disruption everywhere else, but in their own space, when you ask, they always would say, 'oh, this is more of an art, not a science.’
We thought that, just like we were funding companies that are disrupting other verticals, software and data and systems could be applied to our industry, as well. We've always thought that the way to apply it, though, was not to displace humans. We thought of AI as augmented intelligence, not as artificial intelligence. And we always thought that what wins in our space is the marriage of the best of both the human intelligence and the software and data intelligence. When we started out in 2013, it was very much a contrarian view that you could apply data and systems to software. Fast forward to today, it's almost become the opposite — if you don't claim to be applying data to your business, you're considered obsolete.
Where do you draw this data from?
Farmer: This is not like Facebook or LinkedIn or those types of data sources where you're putting data in fields. It's more of like Google, where you're going out to the world to discover websites and apps and products that you didn't even know exist that are constantly, dynamically changing at all times. This is very much like a search function, and 90% of the work is actually what we would call data janitorial work, which is building pipelines, collecting that data, cleaning it up, structuring and then joining the data together so that you can create a mosaic.
Kirnos: Think of us like a mini Google with a Bloomberg terminal. Google goes out and scours the public internet for all kinds of data. It looks at websites, it pulls in images, it pulls in books, it pulls in travel information, and you can search across all those silos of data in one place. We're going out and ingesting all that siloed information, whether it's website information, app store information, so on and so forth. A lot of these things are out in public. Some of these things are paid datasets, just like Google will pay to get financial data from stock exchanges to pull up stock tickers. We do the same thing, and bring it all into one place.
Why not just use PitchBook and CrunchBase or something else?
Farmer: If you combined all of those together, we're probably tracking two orders of magnitude more companies that make it to those in the first place. It's something like maybe a million companies versus 80 million entities that we're tracking. Secondly, the amount of data that's in there is extremely stale. Sometimes it's user generated, so it's also erroneous very regularly, and it's incredibly sparse. How much money they raised, that's a trailing indicator. We're an early-stage seed investor. So, by the time it's in PitchBook or CrunchBase, almost by definition, that's like a tombstone on the thing we missed. The game is over and we lost.
How you approach cleaning up and analyzing the data and correcting for biases?
Kirnos: Biases come in all kinds. We try to look at objective factors like what is the company's ranking in the App Store? How many downloads does it have? How many visitors to your website? What is your revenue? Those kinds of signals. And there can be biases in the datasets that we buy. We're not looking at 100% of your audience, and maybe the audience that we're looking at is more in the urban centers and we're ignoring more of the rural suburban areas. We try to correct for those kinds of biases.
One of the ways that we try to triangulate is by looking at public companies when they report their revenue. We see how close are we able to predict what their revenue is? And we use that to correct our own panels of data. We try to basically unscrew all these things to make sure that we're getting a true representation of the overall audience that might be buying a good or a service or using a certain app or whatever it might be.
How much has Beacon evolved over the past 10 years?
Farmer: It started with the App Store, then talent was the next big thing. Before I started the firm, I interviewed over 500 founders and 170 funds from quant to accelerator to venture to corporate to every permutation to understand how they did competitive intelligence at scale. And there was no one in the venture industry doing it. And just because we have good data, it doesn't mean that's the right place to invest. You have to start with your investment thesis and where you think the right place to invest is and then work the data backwards to figure out who those companies are, and how to track them. Not the other way around.
On the first run, my Amazon Web Services bill was half my management fee. The math didn't work. We had to get super creative and had corporate customers and figured out ways to sell the data.
How does Beacon work for your portfolio companies?
Farmer: We built a Chrome extension that overlays on LinkedIn, because LinkedIn is an amazing website for recruiting, but it doesn't have rankings of people's engineering quality. It doesn't create doppelgangers where you can put a person in and we'll show you 50 people that look just like them. It doesn't understand the facts — like, was this person venture backed? Or what's their personal phone number or email? You can't be like, who were the top ranked AI engineers in San Francisco that have between four and six years of experience and have worked at a seed-stage venture backed company and are likely to look for a job in the next 90 days? There's no place on the earth you can do that search aside from us. We look at the mosaic around that person and now we can give it to every recruiter of every company (in our portfolio). We train them on how to do interviews, how to build a team, how to recon all the processes around recruiting, and then we arm them with sonar to know where to fish, when to fish and with whom.
How do you handle all the sensitive demographic data in Beacon to prevent discrimination?
Farmer: We originally did not capture any demographic data, because we didn't want to show any bias from those things. The problem is, there's been structural inequity in the world since time immemorial. So, the rate of promotion may have inherent human bias in that. The fact that we track promotion might inherently infuse that bias into a system. We've tried very hard not to even give it the data that would allow for any type of bias, but we also recognize that the world is structurally biased. Therefore, we need to do things to proactively counter bias by elevating diversity candidates and things like that to bring them to the fore.
There are some things that are very neutralizing — very much on the merits, like your open-source contributions, your patent contributions, share performance, usage, adoption, etc. And we then blend it on the recruiting side with diversity initiatives and ways to help infuse that from the earliest days of the startups. What you don't want to do is start when a company has 100 people and now you're trying to recruit diversity candidates but you have no diversity at your company. It's very hard to unwind the culture, and you want to do that from day one of a company, which is why we start at the seed stage and think this is so important.
And when it comes to the employees you track in Beacon, what proportion are in the US versus global?
Kirnos: We invest mostly in the U.S. as a fund. So, I'd say the vast majority of the data that we have is relevant to the U.S. There's a lot of startup activity in places like India or Israel and certainly in some European countries. We will selectively get data on those, as well, because those are markets where we can invest and have invested in the past.
Why is it not good to just let AI take the driver's seat?
Kirnos: The short answer is the state-of-the-art technology — machine learning and AI — is just not there yet to be able to do the job. If you let the AI make its own decisions, and you’ll lose a lot of money. It needs guardrails, and the guardrails are provided by the human investment team. Computers are really good at spotting trends and following dots and connecting them into lines. But the underlying meaning and significance of that trend is still something that humans have to interpret.
And the way that we think about leveraging data is to let computers do what they're good at, and then present that information to our team in a highly explainable format. One of the things that maybe you're seeing with (OpenAI’s) GPT is, when it gives you a wrong answer, it's really unclear why. It will be confidently wrong about something and then when you ask it, well, how did you get that? Where does that come from? How do you fix that? How do you debug that issue? It's really difficult.
We have taken an approach consciously. Our machine learning approaches are highly explainable, such that if the machine presents you with a decision, it's easy to understand why that suggestion is being made. And that has two consequences. One, if it makes a mistake, it's easy for us to go back and see where it went wrong and fix it. Second, it creates trust with our team to say, it's not just some black box that's throwing out these predictions and I don't know why. It looks more credible.